Reliable Vaccine Distribution Through S&OE
For anyone that wants to understand the difference between S&OP and S&OE, all they need to do is to listen to the news to hear the latest on the effort for the country to effectively distribute Covid-19 vaccines. There are predictions as to when 70% of the country will have a vaccine, and stories of people waiting in lines, then being told that the vaccine supply did not show up that day and they should come back later in the week. These two different but related predictions highlight the deficiency of a purely S&OP approach vs. when it is augmented with S&OE capability.
With a typical representation at the S&OP level you miss your target for the number of people who are supposed to be vaccinated by the end of March. With an inferior S&OE model you have states that take a bunch of appointments for people to get vaccinated on (say) March 25th, then have to cancel half of them because the vaccine has not arrived. It is in the warehouse!
It correlates to a company figuring out what markets to serve in what quantities in the S&OP plan, then being sure that the deliveries on orders will be on time with the S&OE plan.
An accurate digital model of the supply chain is required to not only support S&OE but also to make accurate aggregate models at the S&OP level that understand averages and variability. It is easy to see the need for the detail at the S&OE level but, what amount of detail is required for S&OP, and what is the cost of having too much detail? A good S&OP model needs to have enough detail to understand the key constraints considering the variability that will be realized at the order level. If you put your hand on a hot stove, and then into a freezing ice bucket, based on average temperatures your hand is ok, but you are not going to be doing very well. With Adexa Digital Blueprint, the supply chain model will have enough level of accuracy needed, so that supply and resources variability can be considered when creating the aggregate model. The cost of too much detail, is the inability to see the forest for the trees. Picture many planners with detail spreadsheets unable to keep up with the changing conditions, and the time required to make a decision.
As an example, you can use the purchase of key materials to see how the S&OP and S&OE models can work together. Adexa Digital Blueprint gives the S&OE model individual Purchase orders each with a due date and arrival date that happen during a particular time of the year under specific weather conditions. In the extended time horizon, the S&OP model would ignore individual orders and plans the same product using average lateness and variability. The problem is that most S&OP systems have very poor representations at any level of planning for both material and capacity. They do a poor job of making correct predictions at the high level and generate plans which are not realistic. One final point is that S&OE and S&OP should not be separate systems; one completes the other. In other words, with the right level of granularity in data, S&OP takes the characteristics of S&OE. An evolutionary process.
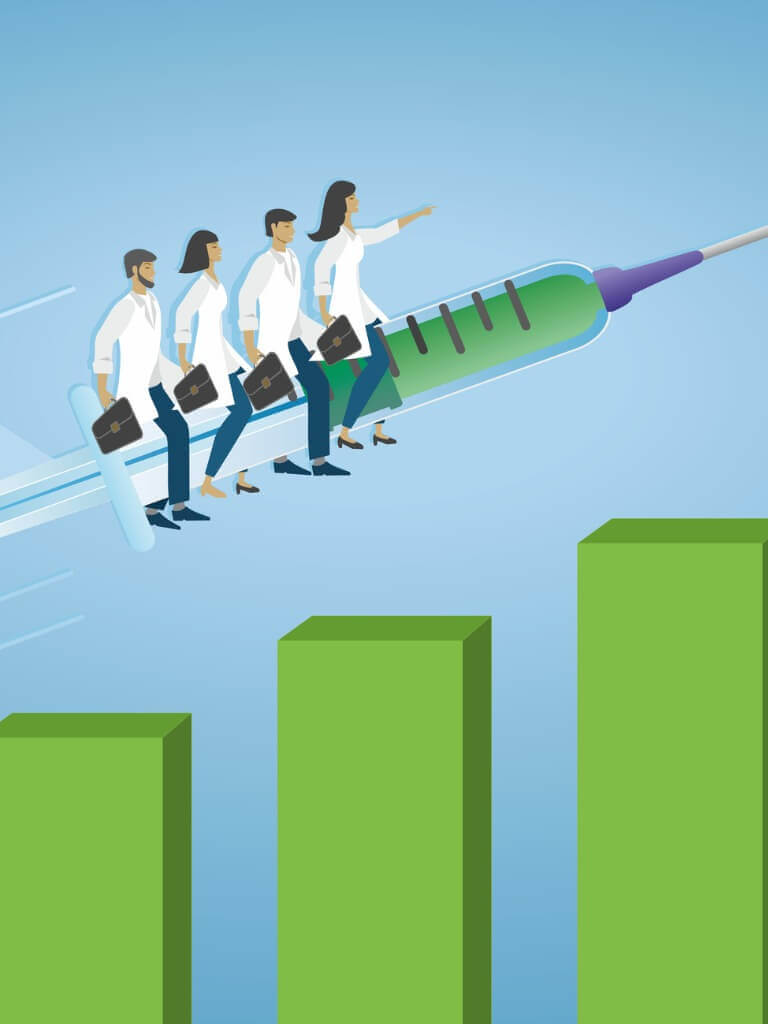